Ronald Ashri, Co-founder & Chief Product and Technology Officer at OpenDialog, and Fawaz Hassan, Solution Architect at Robiquity, combined their expertise in a webinar where they discussed the impact of ChatGPT and Large Language Models on Enterprise Conversational AI.
The discussion covered 6 parts:
- How we arrived at this new era of ChatGPT
- What can large language models do?
- The limitations of large language models
- Its implications for the future of Enterprise Conversational AI
- The challenges and opportunities of implementing large language models
- How we can use large language models like ChatGPT
So, what were their thoughts, opinions and expert insights?
How did we get to this new era of ChatGPT?
Ronald Ashri discussed how large language models such as ChatGPT came to be.
The start of large language models can be traced back to a paper published by Google in 2017, which described a new neural network architecture called Transformers that could efficiently solve language problems better than previous architectures. This allowed large language models to scale more easily, be easily parallelized, become faster and easier to manage.
Since 2017, large language models were trained with more data and processing power, and there have been significant large language models released by companies such as OpenAI, Google, and Facebook. The first GPT model was released in 2018 with 110 million parameters, and in 2019, the second GPT model was released with 1.5 billion parameters. However, when OpenAI released GPT2, they didn’t make it available for public use due to concerns about its power. In 2020, GPT3 was released with 175 billion parameters, and interesting things started to happen at this scale of complexity!
What can these large language models do?
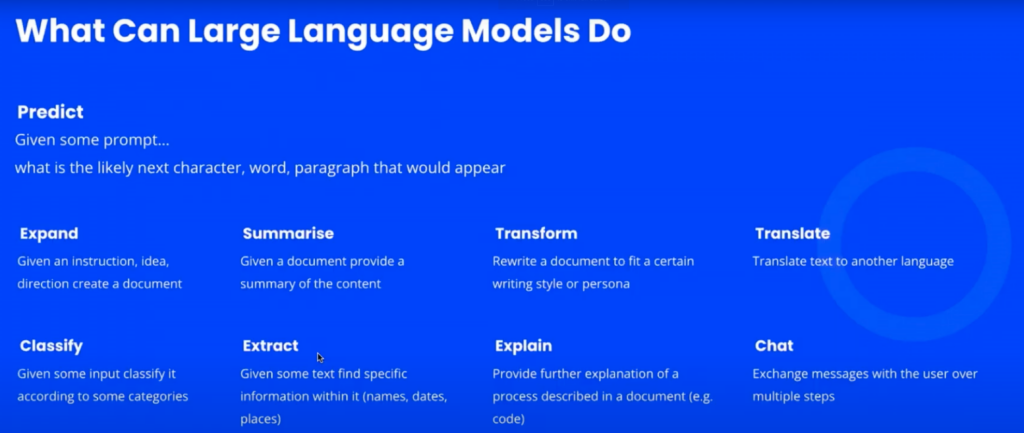
Understanding the limitations of Large Language Models (LLMs)
LLMs are amazing language calculators, but unlike math calculators they can be wrong – capabilities need to be harnessed carefully.
- Truthfulness – large language models have no concept of truthfulness. Just a deep capability to predict what tokens (characters, words) follow next.
- Bias – large language models will replicate and amplify bias found in the data they were trained on.
- Common sense – no understanding of underlying common sense rules.
Explainability – they cannot explain or provide proper sources of how they came to a specific prediction.
What does all this mean for Enterprise Conversational AI?
Ronald explained that the development of large language models, such as ChatGPT, is a step change that can help solve problems better if harnessed properly. The biggest change is a cultural one, as user expectations around conversational interfaces have gone up, and the entire conversation around chatbots has shifted from discussing failures to discussing successes and possibilities.
Ronald spoke about ‘The Conversational AI Automation Cycle’, which highlights the benefits of introducing automation through conversation, including improved customer experience, reduced operational costs, and better data about customers’ needs, and can lead to additional opportunities to improve the customer experience and reduce costs. He warns that organizations that don’t adopt Conversational AI risk falling behind those that do, as they miss out on the positive reinforcement cycles that Conversational AI can create. Ultimately, Ronald suggests that large language models like ChatGPT make it easier to get started with Conversational AI and meet users’ increasing expectations.
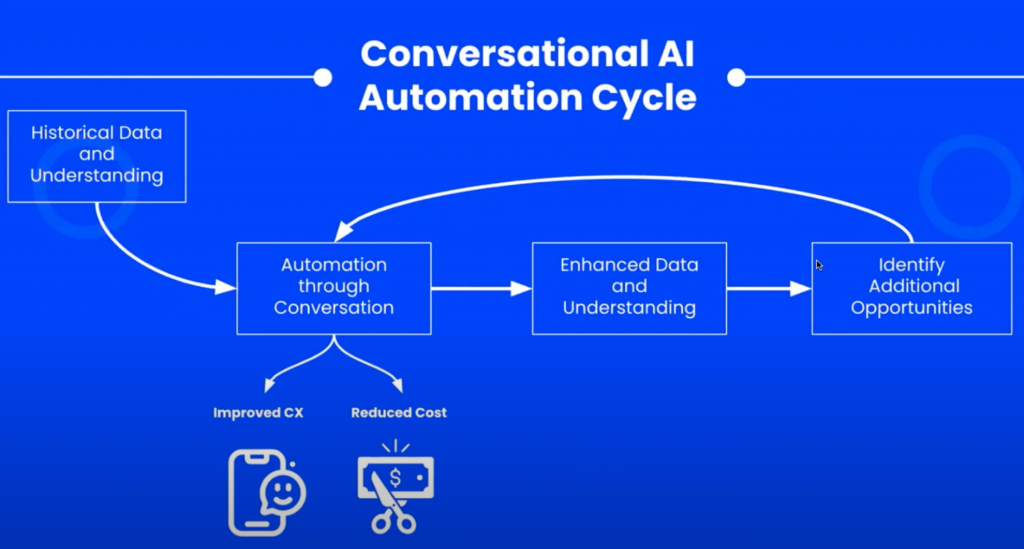
The challenges and opportunities of implementing Large Language Models
Although ChatGPT itself is still not available as a fine-tuned tailored conversational model, Fawaz Hassan spoke about the importance of identifying use cases for integrating large language models into products and the challenges of identification, implementation, scaling, security, and maintenance. He also highlighted the importance of selecting opportunities wisely based on Conversational AI awareness, and understanding where the technology works well and where it doesn’t for your organization.
Use case identification:
Conversational AI Awareness
Knowledge gap in organization on the capabilities and limitations of Conversational AI.
Opportunity Ideation
Once you’ve got a clear picture what sort of business processes could be augmented with Conversational AI.
Measuring ROI
Defining and tracking benefits is key to obtaining buy in and increasing adoption of the technology across the estate.
Opportunities V Not Recommended
Opportunities | Not Recommended |
Customer service support | Highly regulated industries |
eCommerce and online sales | Situations where human empathy is crucial |
Personalized marketing recommendations | Sensitive or confidential information handling |
Data capture and processing |
Large Language Models are growing rapidly, with an estimated 75 million users in one month alone. The expectation is that this growth will continue, making it essential to start looking at use cases now.
How can we use Large Language Models like ChatGPT?
In the context of a Conversational AI platform, using large language models like ChatGPT can be incredibly useful in various phases of the process. According to Ronald, the first phase is conversation design, where a designer thinks about appropriate conversations to have and the context in which they will take place. In this phase, ChatGPT can be used for ideation by generating sample dialogues and providing supplementary data. Additionally, ChatGPT can transform text to make it more suitable for a specific audience, such as using language that is more appropriate for Gen Z.
The second phase is natural language understanding, where the Conversational AI platform integrates different interfaces and back-end capabilities to understand and interpret user input. Here, large language models like ChatGPT can be used to improve the accuracy and effectiveness of the platform’s natural language processing capabilities.
Finally, in the testing and analytics phase, large language models can be used to generate synthetic data for testing and training the Conversational AI platform. This can help ensure that the platform is performing optimally and meeting the needs of its users.
Overall, the use of Large Language Models like ChatGPT, can greatly enhance the capabilities of a Conversational AI platform by improving conversation design, natural language understanding, testing and analytics.
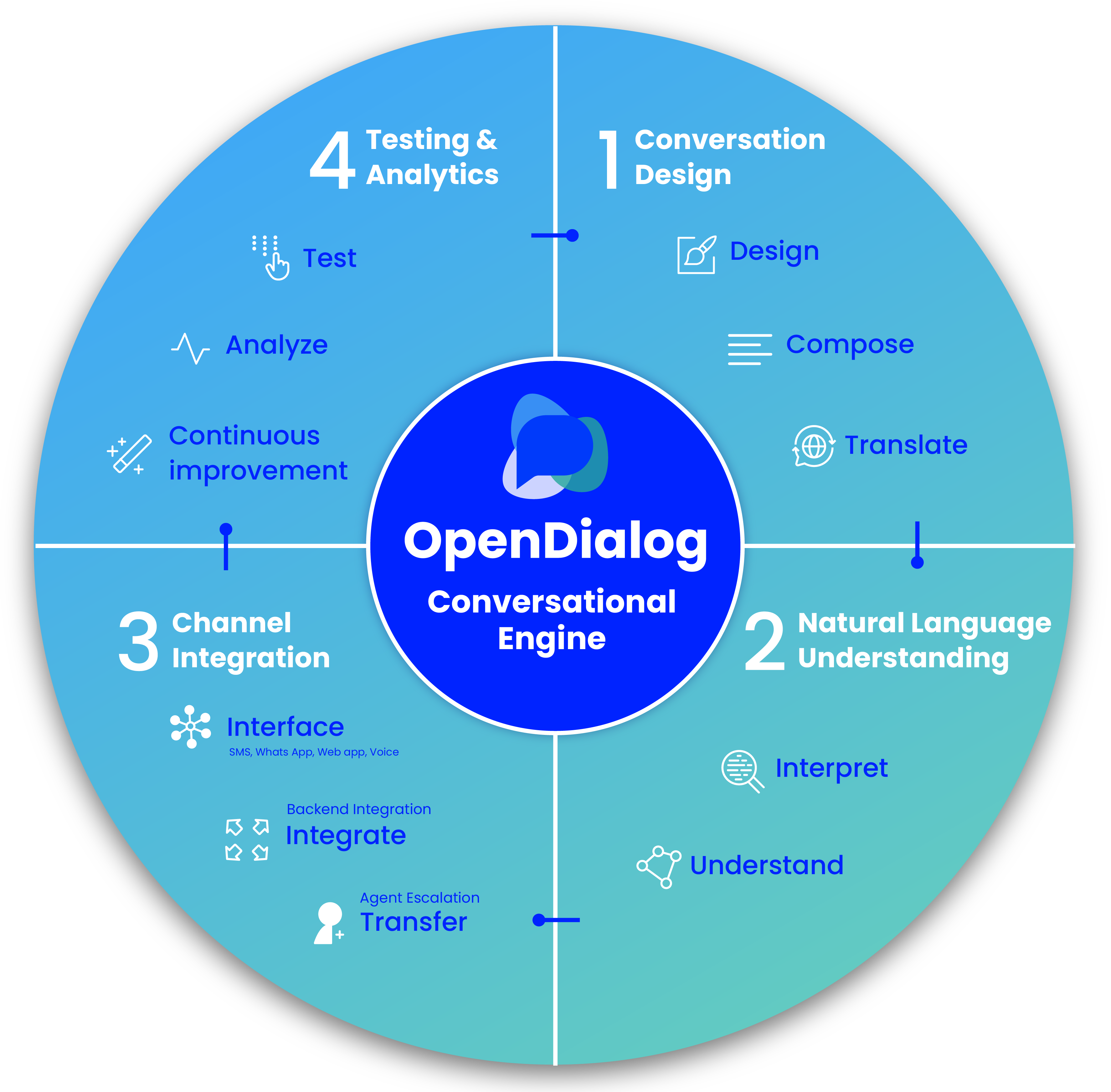